- Topics
- /
- Event-driven servers
- &Architectural patterns
- /
- Maximize the value of realtime analytics with Kafka and Ably
Maximize the value of realtime analytics with Kafka and Ably
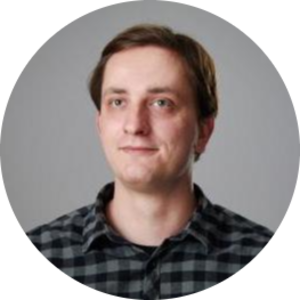
In this blog post, we look at how you can use Kafka and Ably to engineer a trustworthy, high-performance analytics pipeline that connects your backend to end-users at the network edge in realtime. Along the way, we will answer the following questions:
How do you connect your Kafka analytics pipeline to end-users?
How do Ably and Kafka work together to power realtime analytics?
What does an eCommerce analytics architecture powered by Kafka and Ably look like?
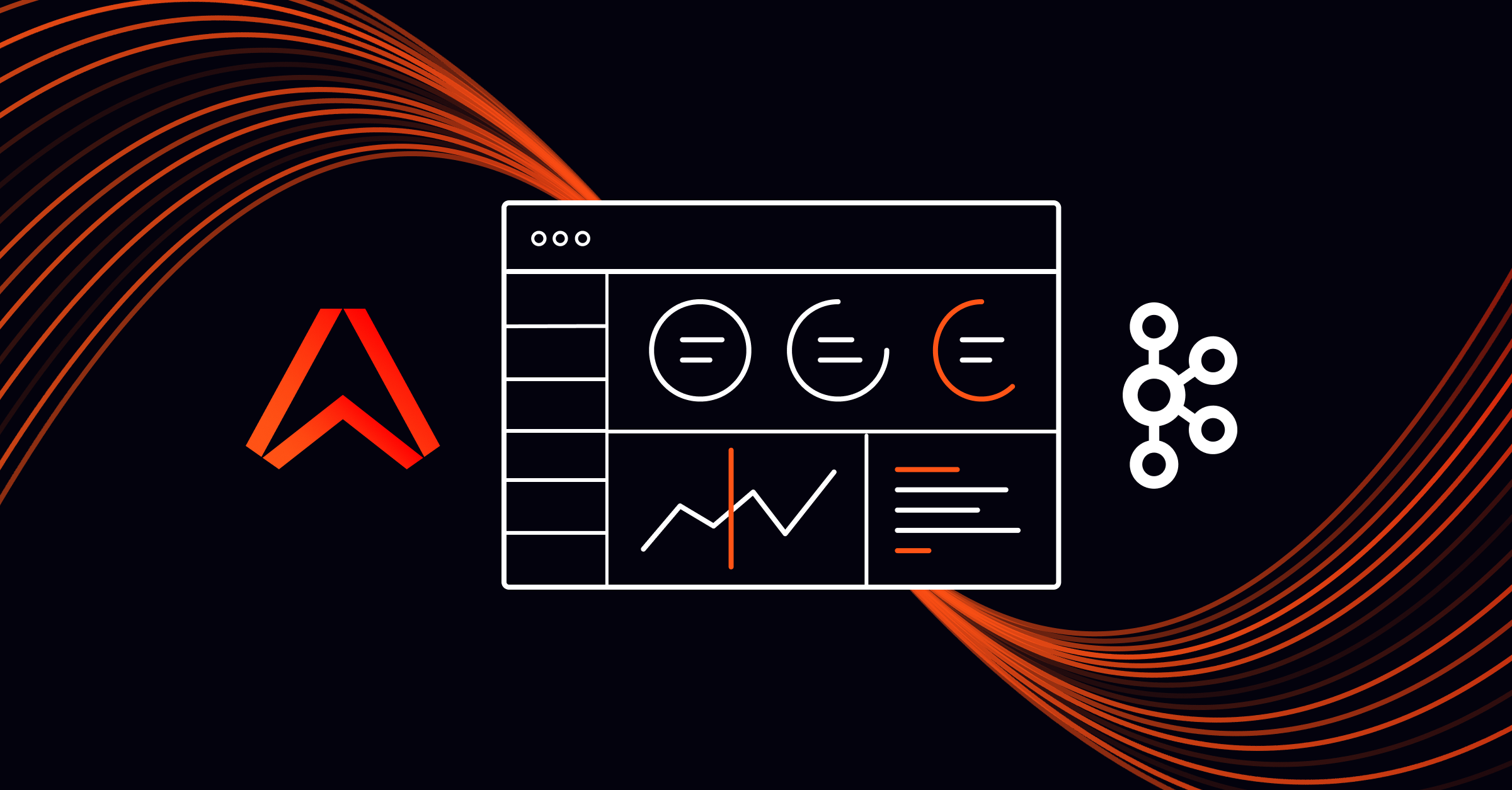
Why do we need realtime analytics?
Realtime analytics (also known as streaming analytics) refers to collecting, storing, and continuously analyzing streams of fast-moving live data, to drive business value. Common examples of use cases powered by realtime analytics include:
Live BI dashboards.
Monitoring financial transactions in realtime to detect fraud.
Realtime anomaly detection for IoT devices.
Analyzing user activity to enhance eCommerce shopping experiences with realtime pricing, promotions, and up-to-the-minute inventory management.
Realtime analytics enable businesses from any industry to gain insights quicker and react faster, increase operational efficiency, reduce costs while creating new revenue, and improve customer experience. In other words, realtime analytics can help organizations gain a competitive advantage.
If you plan to augment your business with realtime analytics capabilities, one of the most important decisions you’ll have to make is what technologies to use. And here’s where Apache Kafka comes in.
Why is Kafka a good choice for realtime analytics?
Kafka is a widely adopted backend pub/sub solution used for event streaming and stream processing. It provides a framework for storing, reading, and analyzing streams of data in realtime. Kafka’s suitability as a reliable, scalable analytics pipeline has been proven for more than a decade; in fact, Kafka was initially designed at LinkedIn to facilitate activity tracking, and collect application metrics and logs.
Let's now briefly summarize Kafka's key concepts: events, topics, and producers/consumers.
Events
Events (also known as records or messages) are Kafka's smallest building blocks. An event records that something relevant has happened. For example, a user has added an item to their online shopping cart, or someone has booked a consultation with a doctor. At the very minimum, a Kafka event consists of a key, a value, and a timestamp. Optionally, it may also contain metadata headers. Here's a basic example of an event:
{
"key": "John Doe",
"value": "Has added item123 to their cart",
"timestamp": "Apr 20, 2022, at 7:45 PM"
}
Topics
A topic is an ordered sequence of events stored durably, for as long as needed. The various components of your backend ecosystem can write and read events to and from topics. It's worth mentioning that each topic consists of multiple partitions. The benefit is that partitioning allows you to parallelize a topic by splitting its data across multiple Kafka brokers. This means that each partition can be placed on a separate machine, which is great from a scalability point of view since various services within your system can read and write data from and to multiple brokers at the same time.
Producers and consumers
Producers are services that publish (write) to Kafka topics, while consumers subscribe to Kafka topics to consume (read) events. Since Kafka is a pub/sub solution, producers and consumers are entirely decoupled.
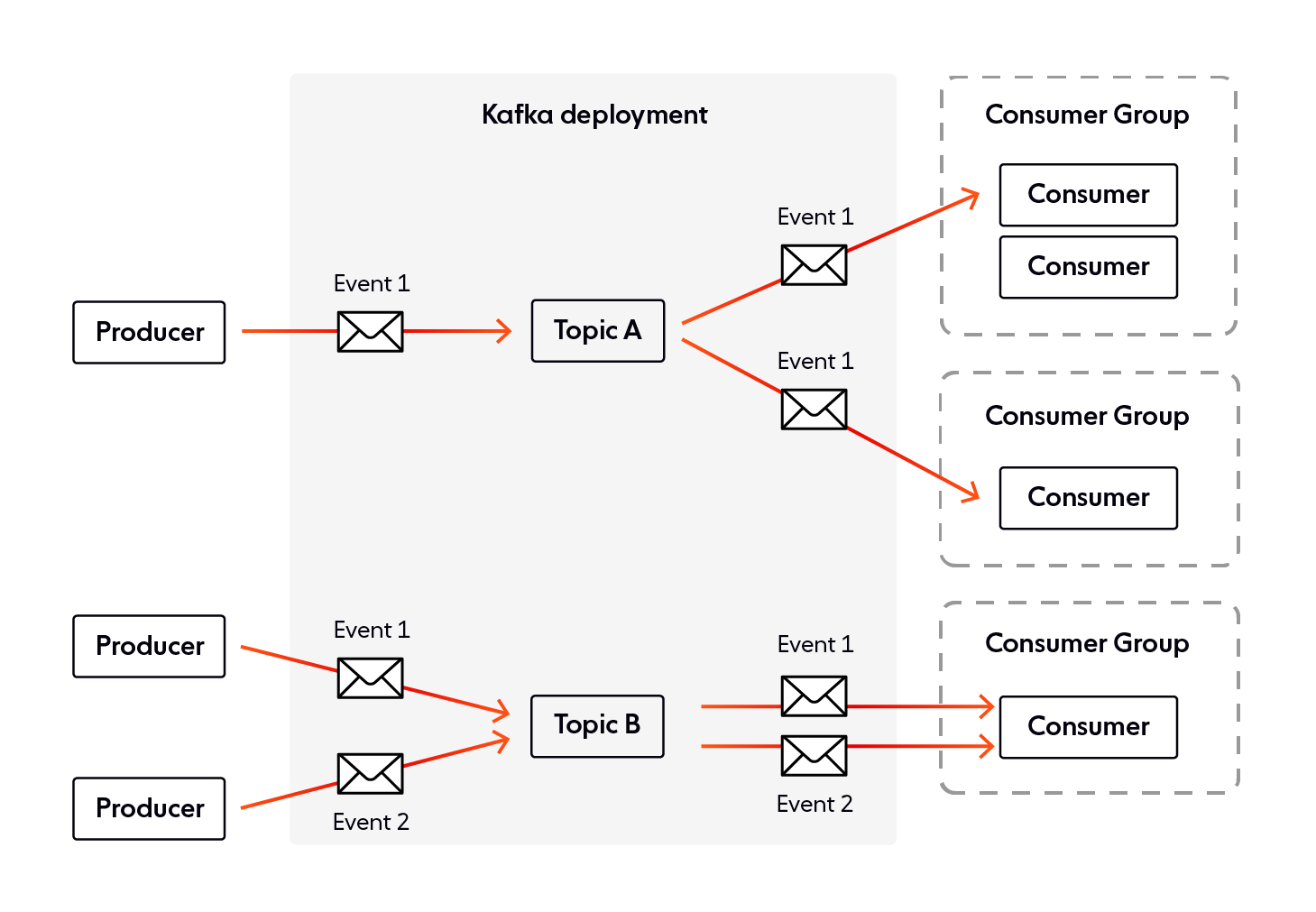
Producers and consumers writing and reading events from Kafka topics
The wider Kafka ecosystem
To enhance and complement its core event streaming capabilities, Kafka uses a rich ecosystem, with additional components and APIs, like Kafka Connect, Kafka Streams, and ksqlDB.
Kafka Connect is a tool designed for reliably moving large volumes of data between Kafka and other systems, such as Elasticsearch, Hadoop, or MongoDB. So-called “connectors” are used to transfer data in and out of Kafka. There are two types of connectors:
Sink connector. Used for streaming data from Kafka topics into another system.
Source connector. Used for ingesting data from another system into Kafka.
Kafka Streams enables you to build backend apps and microservices, where the input and output data are stored in Kafka clusters. Streams is used to process (group, aggregate, filter, and enrich) streams of data in realtime.
ksqlDB is a database designed specifically for stream processing apps. You can use ksqlDB to build applications from Kafka topics by using only SQL statements and queries. As ksqlDB is built on Kafka Streams, any ksqlDB application communicates with a Kafka cluster like any other Kafka Streams application.
What are Kafka’s core capabilities?
Kafka is designed to provide robust capabilities, suitable for engineering realtime analytics pipelines you know you can rely on:
Low latency. Kafka provides very low end-to-end latency, even when high throughputs and large volumes of data are involved.
Scalability& high throughput. Large Kafka clusters can scale to hundreds of brokers and thousands of partitions, successfully handling trillions of messages and petabytes of data.
Data integrity. Kafka guarantees message delivery & ordering and provides exactly-once semantics (note that Kafka provides at-least-once guarantees by default, so you will have to configure it to display an exactly-once behavior).
Durability. Persisting data for longer periods of time is crucial for certain use cases (e.g., performing analysis on historical data). Fortunately, Kafka is highly durable and can persist all messages to disk for as long as needed.
High availability. Kafka is designed with failure in mind and failover capabilities. Kafka achieves high availability by replicating the log for each topic's partitions across a configurable number of brokers. Note that replicas can live in different data centers, across different regions.
How do you connect your Kafka pipeline to end-users?
Kafka is a crucial component to having an event-driven, time-ordered, highly available, and fault-tolerant space of data. A key thing to bear in mind - Kafka is designed to operate in private networks (intranet), enabling streams of data to flow between microservices, databases, and other types of components within your backend ecosystem.
Kafka is not designed or optimized to distribute and ingest events over the public internet (see this article for more details). So, the question is, how do you connect your Kafka-powered analytics pipeline to end-users at the network edge?
The solution is to use Kafka in combination with an intelligent internet-facing messaging layer built specifically for communication over the public internet. Ideally, this messaging layer should provide the same level of guarantees and display similar characteristics to Kafka (low latency, data integrity, durability, scalability and high availability, etc.). You don’t want to degrade the overall dependability of your system by pairing Kafka with a less reliable internet-facing messaging layer.
You could arguably build your very own internet-facing messaging layer to bridge the gap between Kafka and users. However, developing your proprietary solution is not always a viable option — architecting and maintaining a dependable messaging layer is a massive, complex, and costly undertaking. It’s often more convenient and less risky to use an established existing solution.
How do Kafka and Ably work together to power realtime analytics?
Ably is a far-edge pub/sub messaging platform. In a way, Ably is the public internet-facing equivalent of your backend Kafka deployment. Our platform offers a scalable, dependable, and secure way to distribute and collect low-latency messages (events) to and from client devices via a fault-tolerant, autoscaling global edge network. We deliver 750+ billion messages (from Kafka and many other systems) to more than 300 million end-users each month.
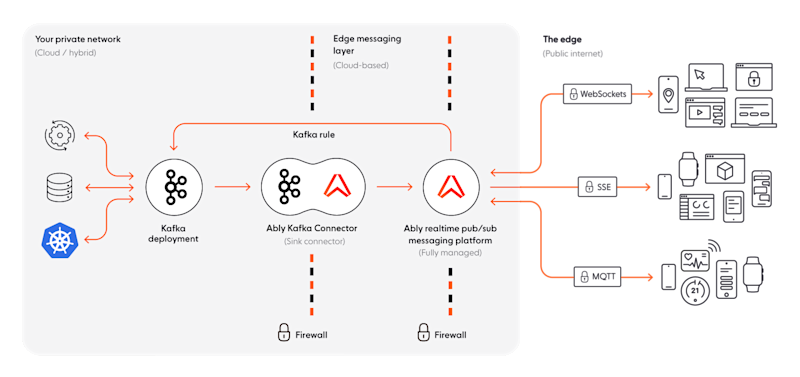
How Ably and Kafka work together
Connecting your Kafka deployment to Ably is straightforward. The Ably Kafka Connector, a sink connector built on top of Kafka Connect, provides a ready-made integration that enables you to publish data from Kafka topics into Ably channels with ease and speed. You can install the Ably Kafka Connector from GitHub, or the Confluent Hub, where it’s available as a Verified Gold Connector.
To demonstrate how simple it is to use the Ably Kafka Connector, here’s an example of how data is consumed from Kafka and published to Ably:
{
"name": "ChannelSinkConnectorConnector_0",
"config": {
"connector.class": "com.ably.kafka.connect.ChannelSinkConnector",
"key.converter": "org.apache.kafka.connect.converters.ByteArrayConverter",
"value.converter": "org.apache.kafka.connect.converters.ByteArrayConverter",
"topics": "outbound:stock updates",
"channel": "stock:updates",
"client.key": "Ably_API_Key",
"client.id": "kafkaconnector1"
}
}
And here’s how a client device connects to Ably and subscribes to a channel to consume data:
const ably = new Ably.Realtime('Ably_API_Key');
const channel = ably.channels.get('stock:updates');
// Subscribe to messages on stock updates channel
channel.subscribe('update', (message) => {
alert(`Left in stock: ${message.data}`);
});
Note that the code snippet above is a JavaScript example, but Ably works with many other technologies. We provide 25+ client library SDKs, targeting every major web and mobile platform. This means that almost regardless of what tech stack you are using on the client-side, Ably can help broker the flow of data from your Kafka backend to client devices.
Additionally, Ably makes it easy to stream data generated by end-users back into your Kafka deployment at any scale, with the help of the Kafka rule.
What are the benefits of using Ably alongside Kafka?
We will now cover the main benefits you gain by using Ably alongside Kafka to engineer a high-performance analytics pipeline that connects your backend to client devices in realtime.
Dependability at scale
Ably augments and extends Kafka’s capabilities beyond your private network, to public internet-facing devices. Our platform is underpinned by Four Pillars of Dependability, a mathematically modeled approach to system design that guarantees critical functionality at scale and provides unmatched quality of service guarantees.
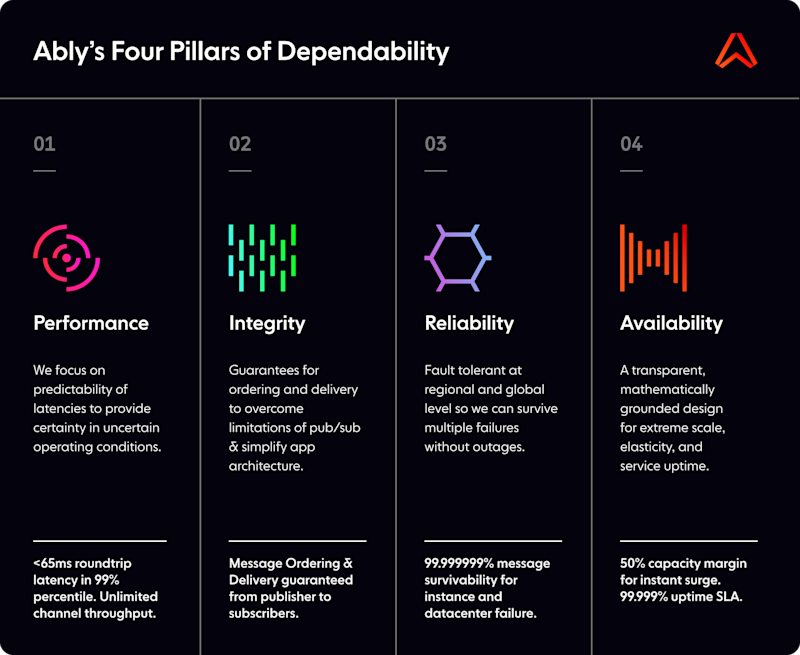
Ably's Four Pillars of Dependability
Flexible message routing and robust security
Since Kafka is designed for machine-to-machine communication within a secure network, it doesn’t provide adequate mechanisms to route and distribute events to client devices.
By using Ably, you decouple your backend Kafka deployment from end-users, and gain the following benefits:
Robust security, as client devices subscribe to relevant Ably channels instead of subscribing directly to Kafka topics. Ably provides multiple security mechanisms, such as authentication and message-level encryption, so your data is always safe. Additionally, our platform is compliant with information security standards and laws like SOC 2 Type 2, HIPAA, and EU GDPR.
Flexible streaming of messages from Kafka topics to Ably channels. This is done with the help of the Ably Kafka Connector. Ably then distributes messages to clients via the closest data center, through the use of latency-based routing.
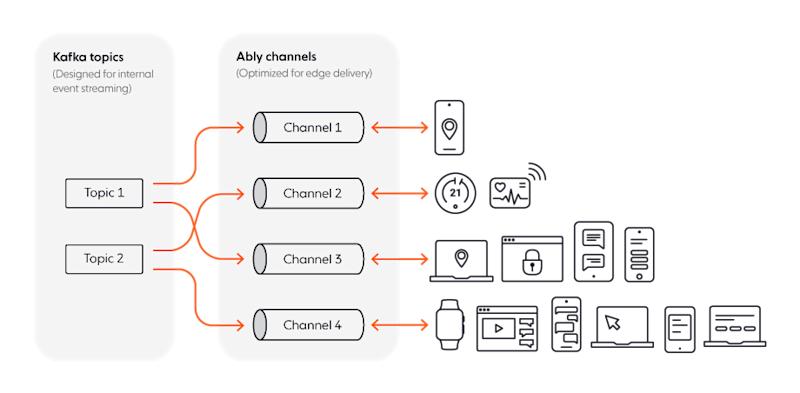
Kafka topics and Ably channels
Scalable pub/sub messaging optimized for end-users at the edge
Kafka works best with a relatively low number of topics, and a limited and predictable number of producers and consumers.
In comparison, Ably channels - the equivalent of Kafka topics - are designed to service an unknown and rapidly changing number of subscribers. Ably can quickly scale horizontally to as many channels as needed, to support millions of concurrently-connected client devices - with no need to manually provision capacity.
In addition, Ably provides rich features that enable you to build dependable realtime experiences for end-users:
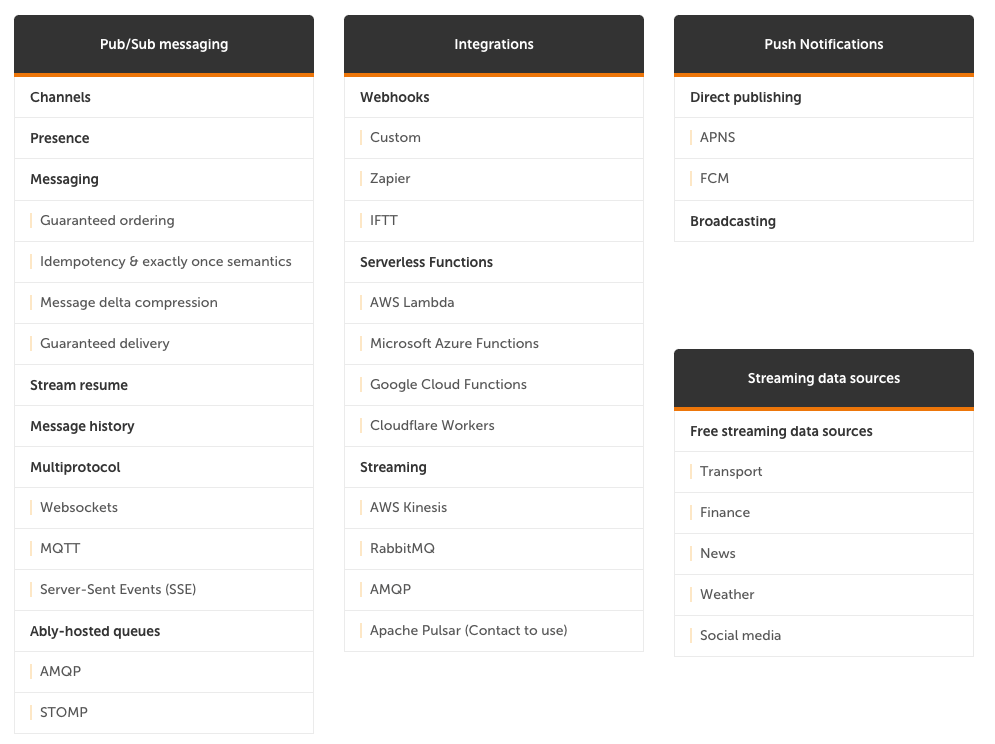
Reduced infrastructure and engineering burden, faster time to market
Building and maintaining your own internet-facing messaging layer to bridge the gap between Kafka and end-users is difficult, time-consuming, and involves significant resources. By using Ably instead, you simplify engineering and code complexity, reduce infrastructure costs, and speed up time to market.
Let’s quickly look at an example, to put things into perspective. Experityprovides technology solutions for the healthcare industry. One of their core products is a BI dashboard that enables urgent care providers to drive efficiency and enhance patient care in realtime - by deploying urgent care professionals and resources according to ‘in the moment’ demand. The data behind Experity’s dashboard is drawn from multiple sources and processed in Kafka.
Experity decided to use Ably as their internet-facing messaginglayer because our platform works seamlessly with Kafka to stream mission-critical and time-sensitive realtime data to end-user devices. Ably extends and enhances Kafka’s guarantees around speed, reliability, integrity, and performance. Furthermore, Ably frees Experity from managing complex realtime infrastructure designed for last mile delivery. This saves Experity hundreds of hours of development time and enables the organization to channel its resources and focus on building its core offerings.
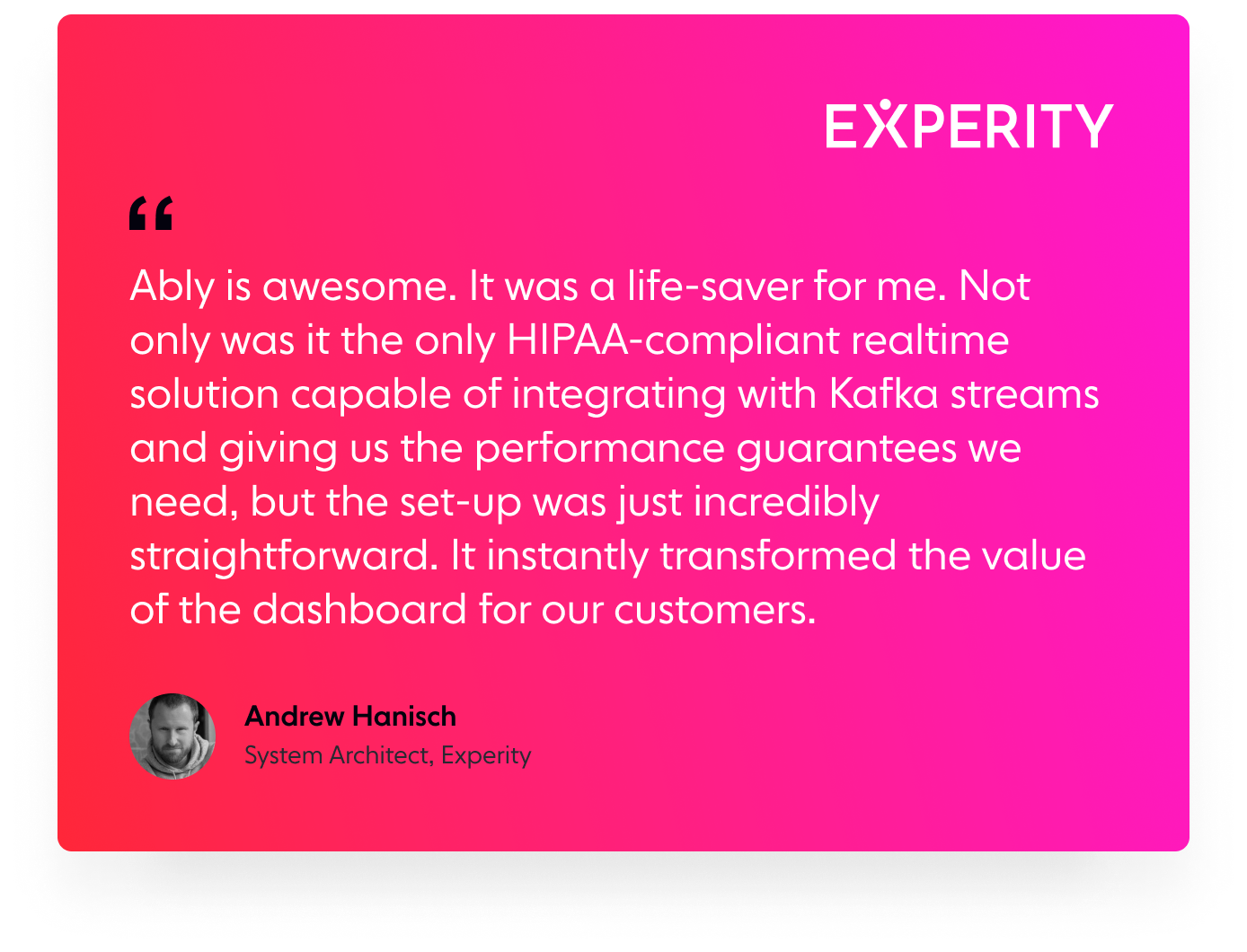
Architecture example: eCommerce analytics with Kafka and Ably
In this section we look at a high-level architecture example to better understand how Kafka and Ably work together, enabling you to build an event-driven analytics pipeline, and react to events as they happen, in realtime.
Our example architecture looks like this:
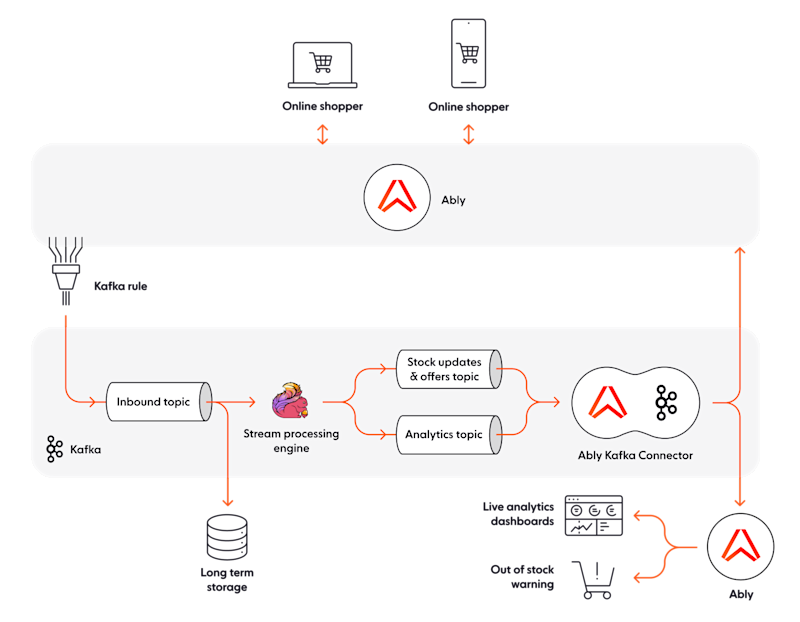
eCommerce realtime analytics powered by Kafka and Ably
Whenever a user buys something or adds an item to their cart, an event (message) is sent to Ably. This stream of events (from all users) is then sent from Ably to an inbound Kafka topic; this is achieved with the help of the Kafka rule.
A stream processing engine then consumes data from the inbound Kafka topic; in our example, we’ve used Apache Flink, a scalable, high-performance solution capable of processing streams of events with low latencies, to extract analytics and insights from raw data. We’ve included Flink to make things more relatable, and because it integrates well with Kafka. However, its use is indicative, not prescriptive. There are other solutions you could use instead of Flink (depending on the specifics of your use case), such as Apache Pinot, ksqlDB, or even a microservice you build yourself.
Once Flink processes the stream of events, the output is written to 2 different Kafka topics: one is used for stock updates & personalized offers, and the other for internal analytics. Data from both these topics is synced to Ably, with the help of the Ably Kafka Connector. Ably then routes data to end-user devices.
While there is a single topic to handle stock updates and offers for all users, you can flexibly shard and route this data to client devices once it’s moved from Kafka into Ably. For example, all users of the eCommerce platform could be subscribed to a “stock updates” Ably channel, and receive realtime updates about the availability of any given product at any point in time. More than that, you can have an Ably channel for each client device, so you can push personalized offers, depending on each user’s activity and shopping habits.
With Ably’s help, data from the analytics topic in Kafka can help the eCommerce provider analyze, understand, and improve business efficiency. For example, you can have live BI dashboards to keep track of things like the number of apples (or any other item) sold, or the number of sales between 1 PM and 2 PM. Or you can receive warnings when you are running low on an item, so you can restock.
Together, Kafka and Ably act as an analytics pipeline that streams and processes events with sub-second latencies, keeping services and end-users in sync in realtime. As both Kafka and Ably are scalable, fault-tolerant, and highly available technologies, a system powered by them can successfully handle unpredictable, fluctuating numbers of online shoppers - even millions of concurrently-connected devices.
Going beyond our architecture example, Kafka and Ably enable you to engineer additional features that are relevant for any eCommerce platform - such as fraud detection and payment processing, or live chat, to ensure first-line support to online shoppers.
What next?
We hope this article has helped you understand the benefits of combining Kafka and Ably when you want to build highly dependable realtime analytics pipelines and related features.
Kafka is often used as the backend event streaming and stream processing backbone of large-scale event-driven systems. Ably is a far-edge pub/sub messaging platform that seamlessly extends Kafka across firewalls. Ably matches and enhances Kafka’s streaming capabilities, offering a scalable, dependable, and secure way to distribute and collect low-latency messages (events) to and from client devices over a global edge network, at consistently low latencies - without any need for you to manage or scale infrastructure.
Although the use case we’ve covered in this article focuses on realtime analytics, you can easily combine Kafka and Ably to power other features and capabilities, such as realtime asset tracking and live transit updates, live score updates, and interactive features like live polls, quizzes, and Q&As for virtual events. In any scenario where time-sensitive data needs to be processed and must flow between the data center and client devices at the network edge in (milli)seconds, Kafka and Ably can help.
Find out more about how Ably helps you effortlessly and reliably extend your Kafka pipeline to end-users at the edge, or get in touch and let’s chat about how we can help you maximize the value of your Kafka pipeline.
Ably and Kafka resources
Recommended Articles
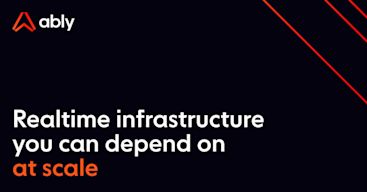
Amazon Kinesis Data Streams
Kinesis Data Streams is a scalable and durable realtime data streaming service.

How to stream Kafka messages to Internet-facing clients over WebSockets
Kafka is used by a plethora of companies to power their realtime data streaming pipelines. Here, we explore how it can be used with WebSockets to stream data over the Internet.
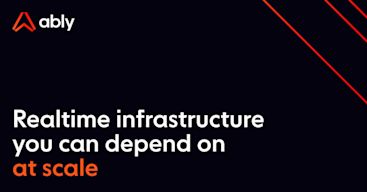
AWS SNS
SNS is a simple, distributed, cloud-native messaging service.